Spss Guide Forecasting
IBM® SPSS® Forecasting enables analysts to predict trends and develop forecasts quickly and easily - without being an expert statistician. Reliable forecasts can have a major impact on your organization’s ability to develop and implement successful strategies. Hp photosmart 735 parts ebay.
Unlike spreadsheet programs, IBM SPSS Forecasting has the advanced statistical techniques needed to work with time-series data regardless of your level of expertise. Analyze historical data and predict trends faster, and deliver information in ways that your organization’s decision makers can understand and use. Automatically determine the best-fitting ARIMA or exponential smoothing model to analyze your historic data.

Model hundreds of different time series at once, rather than having to run the procedure for one variable at a time. Save models to a central file so that forecasts can be updated when data changes, without having to re-set parameters or re-estimate models.
Write scripts so that models can be updated with new data automatically. IBM SPSS Forecasting offers a number of capabilities that enable both novice and experienced users to quickly develop reliable forecasts using time-series data. It is a fully integrated module of IBM SPSS Statistics, giving you all of IBM SPSS Statistics’ capabilities plus features specifically designed to support forecasting. New to Building Models from Time-series Data?
IBM SPSS Forecasting helps you by:. Generating reliable models, even if you’re not sure how to choose exponential smoothing parameters or ARIMA orders, or how to achieve stationarity.
Automatically testing your data for seasonality, intermittency and missing values, and selecting appropriate models. Detecting outliers and preventing them from influencing parameter estimates. Generating graphs showing confidence intervals and the model’s goodness of fit You’re an Experienced IBM SPSS Statistics User? IBM SPSS Forecasting allows you to:.
Control every parameter when building your data model. Use IBM SPSS Forecasting Expert Modeler recommendations as a starting point or to check your work Procedures and Statistics for Analyzing Time-series Data Using IBM SPSS Forecasting with gives you a selection of statistical techniques for analyzing time-series data and developing reliable forecasts. Techniques Tailored to Time-series Analysis IBM SPSS Statistics has the procedures you need to realize the most benefit from your time-series analysis.
It generates statistics and normal probability plots so that you can easily judge model fit. You can even limit output to see only the worst-fitting models - those that require further examination. Automatically generated high-resolution charts enhance your output. Procedures available in IBM SPSS Forecasting include:.
Spss Guide Book
TSMODEL - Use the Expert Modeler to model a set of time-series variables, using either ARIMA or exponential smoothing techniques. TSAPPLY - Apply saved models to new or updated data. SEASON - Estimate multiplicative or additive seasonal factors for periodic time series.
SPECTRA - Decompose a time series into its harmonic components, which are sets of regular periodic functions at different wavelengths or periods.
In the image above, AAAAA through EEEEE are SKU numbers with the relevant monthly sales data provided in the respective columns. There is also a column that indicates the grand total of all SKUs sold in a month (AAAAA +.+ EEEEE + other SKUs not shown in the image above). The last column in the image above reflects the months for which historical sales data are provided.
As with any modeling exercise, we first insert a source node into the modeling canvas. Since our data is in the MicroSoft Excel.xlsx file format, we insert an Excel source node as follows. The Time Intervals node allows you to specify intervals and generate labels for time series data to be used in a Time Series modeling or a Time Plot node for estimating or forecasting. A full range of time intervals is supported, from seconds to years. For example, if you have a series with daily measurements beginning January 3, 2005, you can label records starting on that date, with the second row being January 4, and so on.
You can also specify the periodicity—for example, five days per week or eight hours per day (IBM SPSS Modeler Help). The time interval node is used to specified specify intervals as follows. Lift and Gain Charts are a useful way of visualizing how good a predictive model is.
In SPSS, a typical gain chart appears as follows: In today's post, we will attempt to understand the logic behind generating a gain chart and then discuss how gain and lift charts are interpreted. To do this, we will use the example of a direct mailing company. Let us assume that based on experience, the company knows that the average response rate on its direct mail campaigns is 10%. Let us further make the following assumptions:.
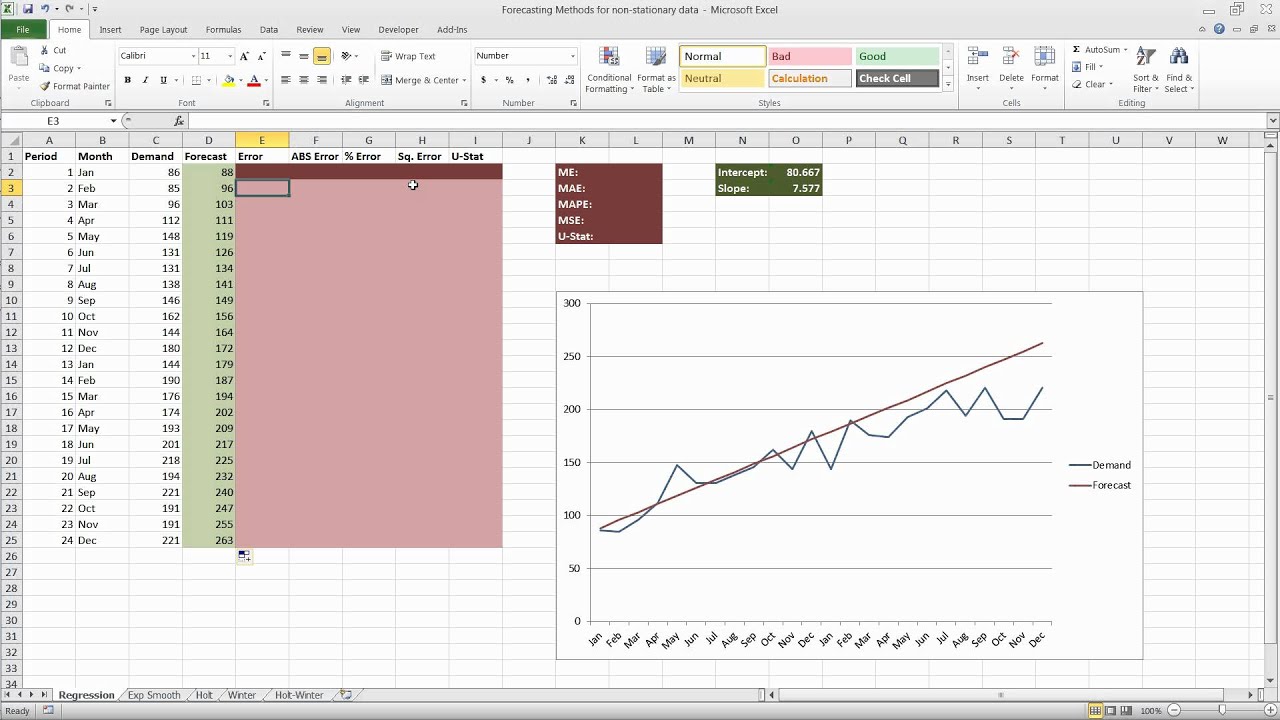
Cost per ad mailed = $1. Return per response = $50 Additionally, let us assume that the company mails out ads in lots of 10,000.
Based on these assumptions, if the company mails out 100,000 ads, a table summarizing the results it would obtain from this campaign is provided below: Now let us assume that the company uses SPSS Modeler to develop a predictive model using data from previous campaigns. 'Response / No Response' is identified as the 'target' fie. Decision Trees are commonly used in data mining with the objective of creating a model that predicts the value of a target (or dependent variable) based on the values of several input (or independent variables). In today's post, we discuss the CART decision tree methodology. The CART or Classification & Regression Trees methodology was introduced in 1984 by, and as an umbrella term to refer to the following types of decision trees: Classification Trees: where the target variable is categorical and the tree is used to identify the 'class' within which a target variable would likely fall into.
Regression Trees: where the target variable is continuous and tree is used to predict it's value. The CART algorithm is structured as a sequence of questions, the answers to which determine what the next question, if any should be. The result of these questions is a tree like structure where the ends are terminal node.